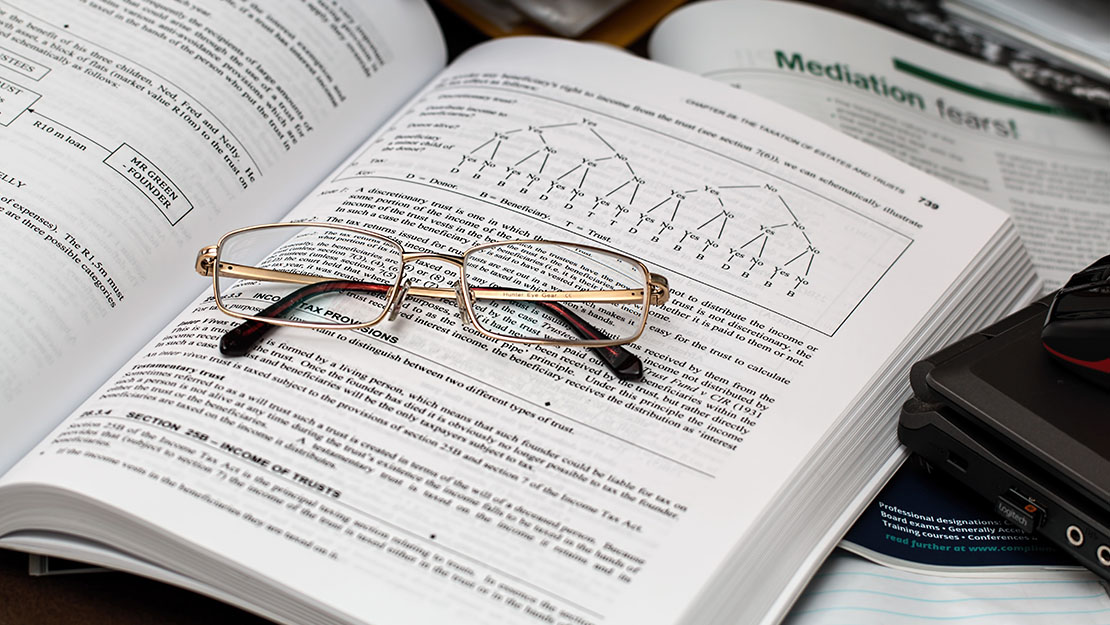
ผู้วิจัย
1. Phajongjit Pijitbanjong 2. Udompong Ketsripongsa 3.Rapeepan Pitakaso 4.Paroon Mayachearw 5.Chaiya Chomchalao
บทคัดย่อ
The purpose of this research was to planning to cultivate economic crops by developing mathematical models and algorithms to solve the problem of planning the selection to find appropriate areas for cultivate economic crops. To consider the economic value for farmers to receive the most profit by using sub-district level information in 8 provinces in the northeastern region of Thailand. The 3 types of economic crops are rice, cassava and sugarcane. This research developed a mathematical model and solved the problem by 3 methods i.e. the LINGO V.11 software, the Difference Evolution algorithm (DE) and Modified Difference Evolution algorithm using the Random best algorithm method (MDE-R). The results from the problem instances categorized into 3 groups showed that testing with small size instances using the LINGO V.11 software and the DE method both gave the same answer but the DE method took less time. When testing with medium size, large size, and case study instances by using the DE and MDE-R methods was found that the MDE-R method gave better answers than DE method at the equal time. The optimization of the highest profit was 12,760,520 baht per cycle.
บรรณานุกรม
[1] Agricultural Information, Bangkok: Office of Agricultural Economics, 2018. [2] Qin, A. K. and Suganthan, P. N. “Self-adaptive differential evolution algorithm for numerical optimization”, In Proceedings of the 2005 IEEE Congress on Evolutionary Computation. p. 1785–1791. Edinburgh, Scotland: IEEE Xplore, 2005. [3] Chakraborty, U. K. and et al. Differential evolution with local neighborhood. New Jersey: IEEE Press, 2006. [4] Josiah, A. and Fred, O. “Differential evolution algorithm for solving multi-objective crop planning model”, Journal of Agricultural Water Management. 97(6): 848-856; June, 2010. [5] Zou, D. and et al. “An improved differential evolution algorithm for the task assignment problem”, Engineering Applications of Artificial Intelligence. 24(4): 616-624; June, 2011. [6] Pitakaso, R. and Thongdee, T. “Solving a multi-objective, Source & Stage location- allocation Problem using Differential Evolution” In Proceeding of the Asoa Pacific Industrial Engineering & Management Systems Conference 2012: p. 1053-1060. Ubon Ratchathani: Ubon Ratchathani University, 2012. [7] Thongdee, T. H. and Pitakaso, R. “Solving a multi-objective, source & stage location-allocation problem: a case study of a bagasse and cassava pulp ethanol plant in northeastern Thailand”, Asia-Pacific Journal of Science and Technology. 17(1): 71-87; February, 2012. [8] Su, Z. and et al. “Multiple emergency resource allocation for concurrent incidents in natural disasters”, International Journal of Disaster Risk Reduction. 17(1): 199-212; January, 2016. [9] Sethanan, K. and Pitakaso, R. “Improved differential evolution algorithms for solving generalized assignment problem”, Expert Systems with Applications. 45(3): 450-459; March, 2016b. [10] Dechampai, D. and et al. “A differential evolution algorithm for the capacitated VRP with Flexibility for mixing pickup and delivery services and the maximum duration route in poultry industry”, Journal of Intelligent Manufacturing. 28(6): 1357- 1376; August, 2017. [11] Qin, A. K. and et al. “Differential evolution algorithm withstrategy adaptation for global numerical optimization”, IEEE Transactions on Evolutionary Computation. 13(2): 398–417; April, 2009. [12] Ketsripongsa, U. and et al. “An Improved Differential Evolution Algorithm for Crop Planning in the Northeastern Region of Thailand”, Journal of Mathematical and Computational Application. 23, 40; doi:10.3390/mca23030040, 2018. [13] Kaewman, S. and et al. “Differential Evolution Algorithm for Multilevel Assignment Problem: A Case Study in Chicken Transportation”, Journal of Mathematical and Computational Application. 23, 55; doi:10.3390/mca23040055, 2018. [14] Srivarapongse, T. and Pijitbanjong, P. “Solving a Special Case of the Generalized Assignment Problem Using the Modified Differential Evolution Algorithms: A Case Study in Sugarcane Harvesting”, Journal of Open Innovation: Journal of Technology, Market, and Complexity. 5, 5; doi:10.3390/joitmc5010005, 2019. [15] Thongkham, M. and Srivarapongse, T. “Improved Differential Evolution Algorithm to Solve the Advertising Method Selection Problem”, Journal of Technology, Market, and Complexity. 5, 61; doi:10.3390/joitmc5030061, 2019.
The purpose of this research was to planning to cultivate economic crops by developing mathematical models and algorithms to solve the problem of planning the selection to find appropriate areas for cultivate economic crops. To consider the economic value for farmers to receive the most profit by using sub-district level information in 8 provinces in the northeastern region of Thailand. The 3 types of economic crops are rice, cassava and sugarcane. This research developed a mathematical model and solved the problem by 3 methods i.e. the LINGO V.11 software, the Difference Evolution algorithm (DE) and Modified Difference Evolution algorithm using the Random best algorithm method (MDE-R). The results from the problem instances categorized into 3 groups showed that testing with small size instances using the LINGO V.11 software and the DE method both gave the same answer but the DE method took less time. When testing with medium size, large size, and case study instances by using the DE and MDE-R methods was found that the MDE-R method gave better answers than DE method at the equal time. The optimization of the highest profit was 12,760,520 baht per cycle.
ความคิดเห็น